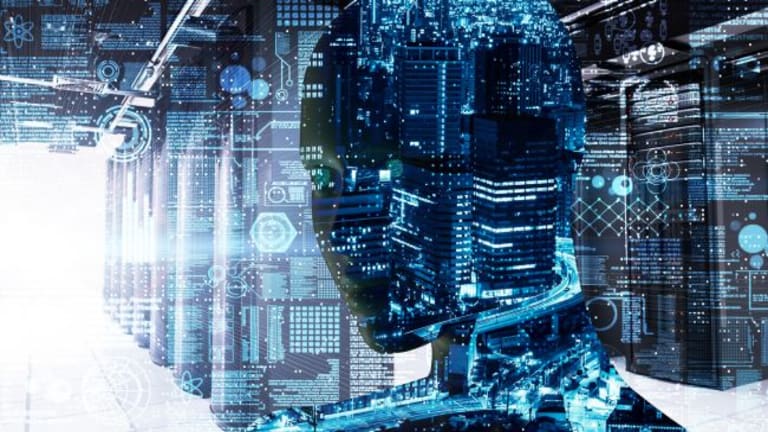
Peter Hinssen on ‘dataism’ and realities of Artificial Intelligence
Technology#Future of Work#WorkTech#Artificial Intelligence
The rise of AI has undeniably reshaped our world, altering how we perceive and interact with everything around us. As we navigate this new landscape, it’s essential to grasp AI’s workings and wield it wisely, unlocking its potential while steering clear of pitfalls. In a keynote presentation during People Matters’ TechHRIN, the famous entrepreneur, author, futurist, and advisor reflected on the concept of the “never normal,” a theme also explored in his book, ‘The New Normal’. He highlighted how data has become the driving force behind our societies and economies, how challenging it was to grasp the full potential of artificial intelligence in its early years, and its unforeseeable necessity in our lives today.
Evolution of AI
Peter reflected on AI’s journey, which began in the 1950s during the Dartmouth conference, where the term 'artificial intelligence' was first coined. In the 1960s, language processing tools like Eliza were invented, and the 1980s saw the rise of machine learning with neural networks, such as those used for character recognition. The 2000s brought deep learning, enabling us to train networks to differentiate between images, such as cats and dogs, with increasing accuracy.
“Over the last decade, the scale and power of AI models have grown exponentially. Today, companies are investing heavily in cloud infrastructure, with projected investments reaching $150 billion this year alone. NVIDIA, a key player in this field, has seen remarkable growth, with a recent quarter generating $26 billion in revenue and nearly $15 billion in profit,” he said.
He also explained how the purpose of AI has evolved from simple language processing to more complex tasks involving vast amounts of data. “Despite periods of excitement and disappointment, known as ‘AI winters,’ progress has continued. We're at a pivotal moment in AI development, similar to the Wright brothers' 1903 aviation breakthrough. We've had the theory and models for AI for years, but only now are we seeing the ‘engine’ powerful enough to make it work. Generative AI has revolutionised creativity, starting with images. Early prompts like "a polar bear playing bass guitar" produced crude results, but quality has since soared.”
Comparing AI's capabilities to dreaming, “using vast amounts of data to generate seemingly real but fictional content. An intriguing example is the phenomenon of AI-generated images of the Pope in a Balenciaga jacket, which isn't true but highlights AI's impact on public perception..The rapid mainstreaming of AI is evident, with technologies like AI-powered Swarovski binoculars and AI-generated summary pendants becoming available.”
AI is transforming but ‘black box’ exists
In the world of work, AI tools like Microsoft Co-Pilot are transforming workflows, from generating PPTs from Word documents to summarising meetings. The landscape of AI is evolving rapidly, driven by companies like NVIDIA, as well as hyperscalers such as Microsoft, which is notably integrating AI into its products; Amazon, which is catching up; Google, which is expanding its AI search capabilities; and Meta, which is pushing open-source AI initiatives.
However, as AI technology advances, challenges such as the black box problem, bias, guardrails, and governance also persists.. “For example, AI sometimes produces erroneous results, like the incorrect identification of the largest cow. Guardrails are in place to prevent harmful outputs, but their effectiveness can vary. Bias remains a significant issue, and understanding AI's limitations is crucial.”
“Training a lot of data means if the input data is biassed, the output will be even more biassed. These tools are incredibly efficient at generating content that reflects the biases present in their training data.”
Will jobs change or disappear?
The biggest challenge in a corporate environment is governance.. “while we were drowning in information, we were starving for knowledge…A new field is emerging: content science. While data science has focused on structured data—analysing numbers and predicting outcomes—content science addresses the vast amounts of unstructured information like PowerPoints, PDFs, Slack channels, and emails. This unstructured data often makes up 70-80 per cent of information within organisations and remains largely unmanaged.”
“Structured data has seen significant advancements over the past 25 years, from analysing past events to predicting future ones. In contrast, unstructured data management has lagged..” The challenge now is integrating LLMs with this unstructured content. This integration, known as Retrieval-Augmented Generation (RAG), combines prompts with your unique content to produce valuable insights. “For example, McKinsey's experience with Lily underscores the importance of RAG. They found that rebuilding their knowledge base and implementing RAG operations were key to success. Moving forward, we may need content scientists, content engineers, and content analysts to make RAG work effectively—new roles we don't fully understand yet.”
This could also address the productivity paradox. Despite using digital tools, many of us are not significantly more productive. “Furthermore, AI might change the nature of work output. For instance, some of your work may range from excellent to mediocre, and AI could potentially eliminate the mediocre content by suggesting better versions before you send them out. However, this could make finding exceptional work more challenging in a sea of improved but average content. Recent developments, like Google's DeepMind achieving high standards in the Mathematics Olympiad, highlight the growing capabilities of AI. Yet, humans still excel in complex, non-linear tasks. Hans Moravec’s paradox illustrates that what we find hard is often easy for computers, and vice versa.
In a world increasingly dominated by AI and algorithms, the role of humans as "framers" remains vital. We are the ones who provide context and critical thinking to shape the situation. As the former CEO of IBM noted, while 10% of jobs might disappear, 100% will undergo change.”
AI and HR: Challenges and Resolutions
From an HR perspective, it's important to approach this with a balanced view. Embracing new capabilities is easy, but removing outdated ones is challenging. As Peter Drucker wisely said, 'If you want something new, you have to stop doing something old.' This is crucial for HR professionals who need to navigate both opportunities and challenges. We face potential cost reductions and growth opportunities, but also risks of market erosion. The future costs of AI technology remain uncertain, adding to the challenge.
For HR, this is a chance to rethink the future of work and become a more strategic partner. The challenges are significant, particularly regarding future skills, work structures, and managing a multi-generational workforce. We need to evolve from data management to advanced analytics, and security issues are becoming more complex. Data leakage was just the beginning; now we’re navigating a new era with unknown costs.
In summary, this is a tremendous opportunity for companies and HR to play a pivotal role. As David Wilkinsford puts it, we’re dealing with the intersection of human challenges and advanced technology."