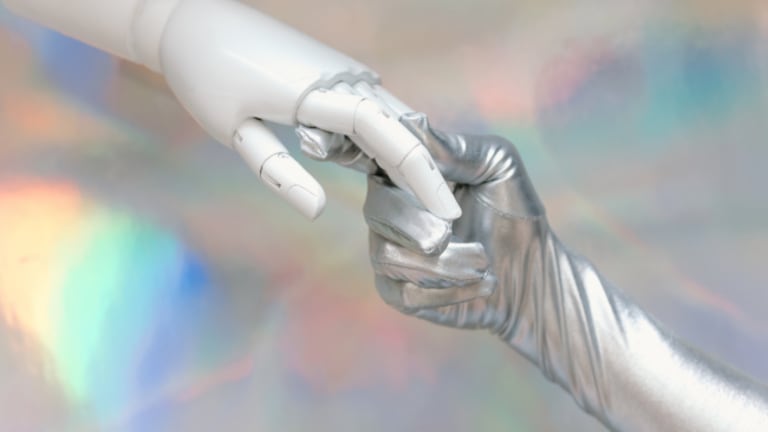
Who gets the credit? Or, how to recognise human work in a marketplace flooded with AI
Employment Landscape#Artificial Intelligence#Future Of Human Capital#EyeonAI
This article was first published in the March edition of People Matters Perspectives.
‘Credit: Google.’
It wasn't so long ago that such references in an essay or project made teachers and managers alike facepalm and then call the perpetrator in for a talk.
Back when Google’s search engine was still new, people cited it as their source from a combination of convenience, ignorance, and on occasion simple laziness. Search was easy, they didn’t understand how the engine worked or why it was important to attribute their source properly, and most of the time it was too much effort to attribute correctly.
But give them some credit. At least most people stated that they had found their content by using Google Search, and didn't attempt to pass it off as their own work.
Today, passing the output of generative AI off as one's own work, without so much as a ‘Credit: ChatGPT’, has taken the place of that ingenuous two-word reference, and for much the same reasons. So…
Why pay a human when the AI can do it?
That question is why Goldman Sachs, in 2023, predicted that generative AI will displace 300 million jobs. Subsequent research in 2024 found that the demand for human workers to fill AI-prone roles dropped by as much as 30% after the introduction of generative AI tools. And the follow-up question, of how to keep humans sufficiently relevant that they can stay employed, has been the subject of global angst for months.
It’s easy to see why AI is touted as the solution to pinched salary budgets. At its best, generative AI output is indistinguishable from the work of highly skilled humans.
And even at its laziest, an uncurated piece of generative AI output can still be mistaken for actual human work - albeit the work of a mediocre high school student utilising purple prose to fill a minimum word count for an essay assignment.
Now think of a poorly operated LLM as having the capabilities of such a student, and immediately we have a few ways to tell that the AI did all the work and the human did nothing.
- Depth of thinking not reflective of the person’s actual capabilities: e.g. someone with 20 years of senior-level experience regurgitating the chapter summaries of a textbook.
- Very broad scope and very shallow depth: e.g. 20 different sub-topics in 800 words, each one given only a few generic sentences, with no elaboration.
- No contextualisation: motherhood statements with lack of data, failure to address any specific issue, no connections drawn to external events or the current environment.
- No underlying thesis: no question posed or answered, no element of discovery or analysis, a general air of ‘words for the sake of having words’.
Such a piece of work might as well be attributed ‘Credit: ChatGPT’, which would certainly be more honest than offering it up as human work. Why pay the human, indeed.
How do you know when the human actually did some work?
Reverse the above list of negatives, and you have the signs of good quality human work: critical thinking, refinement of scope, contextual placement, an underlying argument drawn from real-world experience. Human intervention and curation, properly done, can elevate AI output at every stage of the content creation process from refining the concept to checking the accuracy and relevance of sources, to reviewing and editing the final output.
The only part of content creation where the work can be almost completely foisted off on generative AI is the actual creation stage. In principle, this is no different from the automation of manual labour.
In practice, even simple manual automation needs a pre-existing setup in order to work. Amazon’s famous warehouse automation system, for example, runs on the foundations of an underlying inventory planning system, strict warehouse layouts, labelling system, and others. Omit any of those, and the associated operation stage breaks down.
Creation via generative AI is no different; skip or skimp on the supporting framework, and the output will be just as skimpy.
2023 studies by MIT Sloan and Harvard Business School researchers, later borne out by similar studies in 2024, showed that generative AI improves the performance of less experienced workers by up to 30% and the performance of more experienced, more skilled workers by up to 40%. All studies flagged out one thing in common: the need for cognitive effort, the intellectual equivalent of setting up that supporting framework in which the automated tool can operate.
You may also like:
- Why hiring the right talent takes time & strategic HR investments pay off
- New hiring mantra for onboarding long-term high-performers
- 5 ways (with Insights) to build a more positive workplace culture
- The real cost of hiring and losing the 'right' talent
People’s productivity might initially come from the tool, but for the framework to be of good quality, that cognitive effort ultimately needs to be internalised.
In other words, the human might start off by taking direction from the LLM’s output, but at some point the human must advance enough to be the one giving the AI instructions and curating its work.
That advancement is what we need to value in an AI-powered environment where human cognitive effort and machine patterns become intertwined. It is why we continue to pay full-time employees and not just buy a heap of platform licenses assigned to hourly temps. And it is why, in education or in professional life, we should not settle for ‘Credit: ChatGPT’ any more than we settled for ‘Credit: Google’.
Did you find this article insightful? People Matters Perspectives is the official LinkedIn newsletter of People Matters, bringing you exclusive insights from the People and Work space across four regions and more. Read the January, February , and March 2025 editions here, and keep an eye out for the upcoming April edition rolling-out soon.