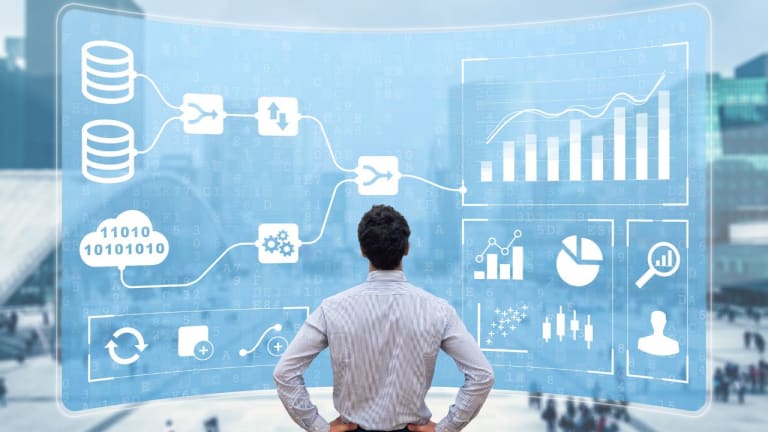
Are AI-driven performance evaluations truly fair?
HR Technology#HRMetrics#DigitalTransformation#DigitalCultureReset#BreaktheBias#Artificial Intelligence
AI-driven performance evaluation systems have revolutionised the workplace, providing data-driven insights into employee productivity, efficiency, and contribution. However, as organisations increasingly rely on AI to assess employees, ethical concerns surrounding fairness, bias, and transparency have emerged.
What raises concerns in AI-based performance evaluations?
While AI has the potential to enhance objectivity and streamline performance tracking, it can also reinforce existing biases, erode employee trust, and lead to unfair consequences. Understanding the ethical implications of AI-based performance evaluations is crucial in ensuring that these systems promote fairness, accountability, and transparency.
AI-driven performance evaluation systems rely on historical data, but if this data contains bias, AI will replicate and even exacerbate those biases. This can lead to unfair assessments that disadvantage certain groups of employees, particularly those from underrepresented backgrounds.
One of the main causes of bias in AI-based performance metrics is biased training data. If past performance reviews include human biases—such as unintentional gender or racial preferences—AI will internalise and perpetuate these patterns.
Additionally, AI models often lack diverse and representative training data, which means they struggle to fairly evaluate employees from different demographics. If the data predominantly reflects the work patterns of a specific group, AI may unfairly favour individuals with similar characteristics.
Sameera Bandara, an Australia-based Cybersecurity Leader writes, "One of the biggest challenges in using AI for any task is that social biases have unfortunately been transferred to the products that we have built." She quoted works from award-winning journalist Tracey Spicer's book 'Man-Made', which discusses the troubling implications of AI-linked social biases.
She added, "In short, your AI solutions are as biased as the training data set. For example, researchers have demonstrated that AI-based facial recognition systems are more likely to falsely identify African Americans over Caucasians as suspected criminals. So, yes a performance evaluation can be affected by an individual's biases but an AI-based performance evaluation can be affected by social biases through the dataset. An AI-based performance evaluation might be ideal when the evaluation is purely numerical."
Another key issue is the AI’s inability to understand nuanced performance factors. Emotional intelligence, leadership qualities, and teamwork contributions are difficult to quantify, meaning AI may undervalue employees who excel in these areas.
Furthermore, AI systems often rely on proxy metrics, such as keystrokes, email frequency, or attendance, to gauge performance. This approach can disproportionately impact employees with disabilities, remote workers, or those with non-traditional work schedules.
A notable example of algorithmic gender-bias occurred with Amazon’s AI-driven hiring tool in 2018, which systematically downgraded resumes that included words like "women’s," as past hiring trends had favored male candidates. This same type of bias can easily infiltrate AI-powered performance evaluations, leading to unfair ratings, missed promotions, and reduced employee morale.
Nadeen Matthews Blair, CEO of CAG Ltd writes, "AI can be used to auto populate scores for objective metrics and for drafting subjective feedback based on recorded coaching mechanisms. HR should leverage AI to help detect bias by evaluating if trends in scores for different underrepresented groups, and to see if there are inconsistencies in application of final scores based on objective data. Language in subjective areas can be reviewed to detect inconsistencies and bias."
Transparency in AI Decisions
Employees must understand how AI evaluates their performance and should have the ability to challenge unfair assessments. Transparency is critical in fostering trust between employees and AI-driven evaluation systems.
One major concern is the "black box" nature of AI models, where employees have no visibility into how decisions are made. If workers do not understand why they received a particular performance rating, they may perceive the system as unfair and arbitrary. To maintain trust, organisations must prioritise AI explainability, ensuring that employees receive clear reasoning behind their assessments.
Transparency also includes allowing employees access to their performance data and understanding the metrics being used.
Organisations should conduct regular AI audits to check for bias, unfair patterns, or unintended discrimination. These audits can help identify disparities in performance ratings across different demographic groups and prompt corrective actions.
Human oversight is equally essential. Managers should not blindly trust AI-generated evaluations but instead use them as a tool to supplement, rather than replace, human judgment. Employees should also have the right to challenge AI-driven assessments through a structured appeals process.
U.S.-based tech leader, Sean Bair writes, "AI can address biased performance evaluations by implementing objective and data-driven assessment methods. Firstly, it can analyse performance metrics across various dimensions, mitigating subjective biases. Natural Language Processing (NLP) algorithms can review feedback comments for fairness and consistency. Additionally, machine learning models can identify patterns of bias in evaluation criteria and suggest adjustments. Moreover, AI-driven dashboards provide transparency and accountability in the evaluation process. By leveraging AI, organisations can ensure fair and equitable performance assessments, promoting a more inclusive and merit-based workplace culture."
Regulatory considerations are another critical factor in AI transparency. Laws such as the EU’s AI Act and GDPR are pushing for greater accountability in AI-driven decision-making, requiring organisations to disclose how AI-generated conclusions are made. Ensuring compliance with such regulations not only protects employees but also helps organisations build credibility and trust in their AI systems.
AI advocate for portfolio management, Roni V explains, "Adopting AI in performance evaluations offers a way to enhance fairness and objectivity, but it requires careful implementation and ongoing oversight. Firstly, employees should understand how their performance is being evaluated and what factors the AI considers. This transparency builds trust in the AI system. Secondly, it’s important to regularly audit and update the AI algorithms to ensure they remain unbiased and relevant to the evolving workplace dynamics. Finally, while AI can significantly reduce bias, it should not entirely replace human judgment. A hybrid approach, where AI-generated insights complement human evaluations, can provide a balanced and fair assessment of employee performance."
You may also like:
- Turning Performance Reviews into a Year-Round Culture of Appreciation
- Rethinking performance measurement in the AI Era
- Remote Work Culture & Recognition: What really works?
- Performance vs Pay: Defining the thin line
Bias mitigation strategies
To ensure fairness in AI-driven performance tracking, companies must actively implement bias mitigation strategies. One of the most effective approaches is conducting regular audits and bias testing. By analysing performance scores across different demographic groups—such as gender, race, and age—organisations can detect and rectify disparities. Disparities should be addressed through model retraining, ensuring that AI systems provide equitable evaluations.
Using diverse and representative training data is another crucial step in reducing bias. AI should be trained on datasets that reflect a broad range of employees and work styles to prevent favoritism toward specific demographics.
Additionally, synthetic data generation and bias correction techniques can help balance representation. Implementing human-in-the-loop (HITL) systems, where human managers validate AI recommendations before acting on them, ensures that AI serves as a support tool rather than a sole decision-maker.
Algorithmic fairness techniques, such as adversarial debiasing and re-weighting models, can further mitigate systemic bias. Organisations should make AI models interpretable and accessible to employees and HR professionals. This ensures that performance evaluations are not only accurate but also comprehensible to those being assessed.
Talking about why the ethics of AI are not optional but essential, Chief AI Officer at FANR Awadh AlMur writes, "Technology should uplift society, not expose it to harm. As AI adoption accelerates globally, keeping its development ethical and responsible is critical."
He added key considerations: "To ensure this, we must address key areas:
- Bias – Train AI with diverse, inclusive data.
- Privacy – Use data transparently and with consent.
- Security – Protect sensitive information with strong safeguards.
- Job Disruption – Equip people with AI-relevant skills."
These considerations should be accounted for wherever AI is implemented in HR systems, whether in training and development, hiring, or performance evaluations and management.
Therefore, 5 ethical considerations in AI-driven performance metrics are:
- AI systems can inherit and perpetuate biases from historical training data, leading to unfair evaluations.
- Employees should have transparency into how AI assesses their performance and be able to contest unfair ratings.
- Over-reliance on AI-driven metrics can lead to an incomplete understanding of employee contributions.
- Organisations must balance AI transparency with privacy concerns, ensuring that AI does not infringe on employee rights.
- Regular AI audits, diverse training data, and human oversight are essential for mitigating bias and ensuring fairness.